Research
The CDS Lab at UT Arlington has interests in a wide range of interdisciplinary scientific research topics, all of which revolve around applications or development of state-of-the-art Data Science Techniques, in multiple stages of data aggregation, reduction, analysis, and scientific inference based on the fundamental principles of Probability Theory.
biomedicine
Biomedicine
Computational Oncology
The ultimate goal in the field of computational oncology is to develop mathematical models that are capable of describing and predicting relevant biological phenomena simultaneously at all physical scales, from sub-cellular to tissue level. Achieving this holy grail, however, is extremely challenging at the moment given the computational expense and complexity of biological phenomena, perhaps comparable to the complexity of bringing fusion power down to earth for everyday energy needs of the society.
Within the field of Computational Oncology, the CDS Lab is broadly interested in understanding and modeling the growth of malignant brain tumors, understanding the physics of cancer metastasis (i.e., how cancer cells spread from the primary tumor to distant organs in the body), as well as developing data-driven methodologies for optimal segmentation and delineation of different tumor cell types in Biomedical Imaging datasets (e.g., MRI, CT). The current research focus is on developing a biophysically realistic models of single cell growth, motility, and eventually, the interactions of cells with their environment and other cells.
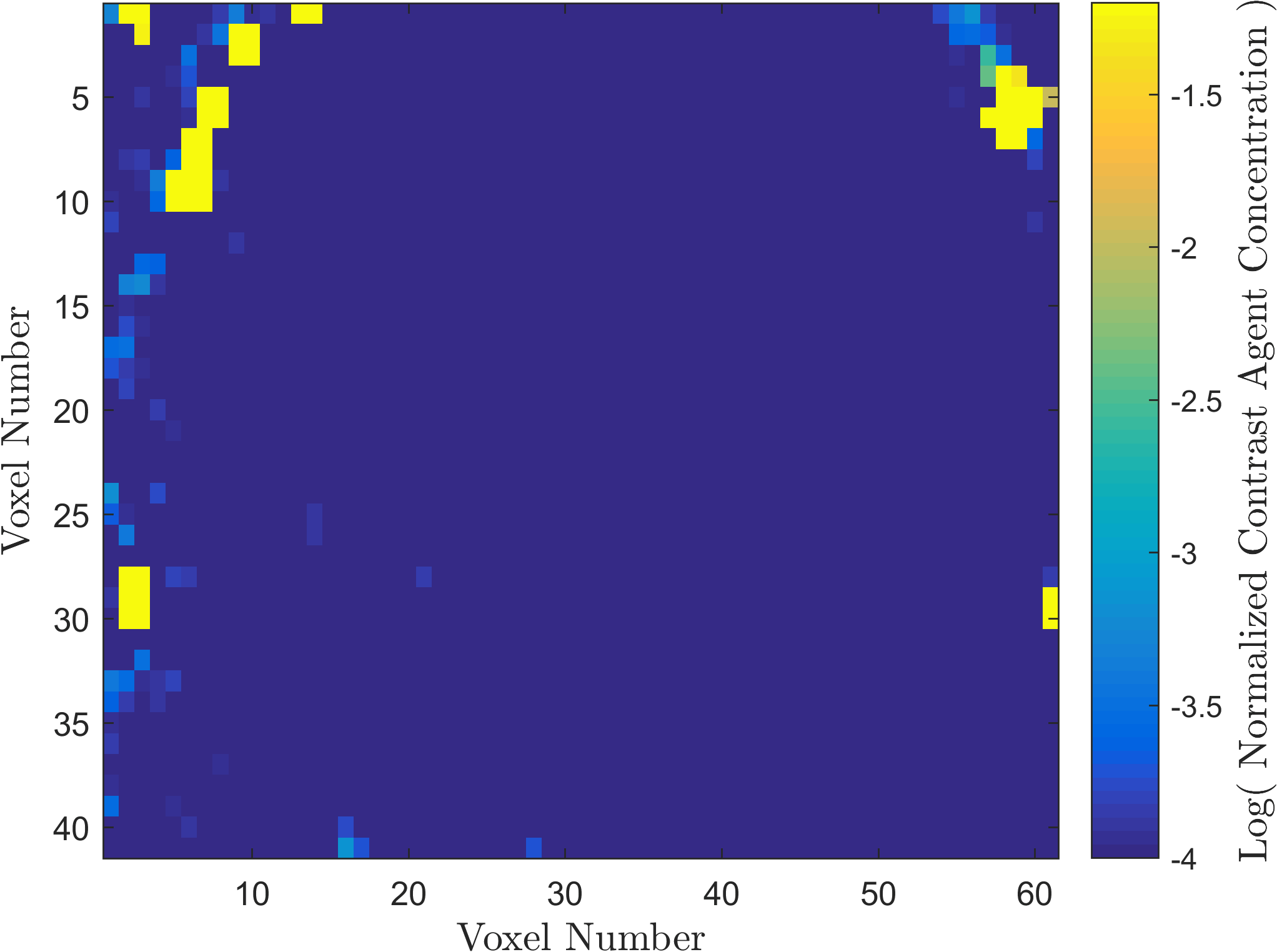
Back to Top
Stroke Lesion Segmentation
Along with Cancer, Ischemic Stroke is one of leading causes of death in the United States and worldwide. One of the significant challenges in the appropriate and timely treatment of ischemic stroke is the identification of the affected region of the brain, that is, the dead tissue resulting from the stroke, as well as the shadow area surrounding the dead necrotic tissue, where the blood flow is restricted, but not completely blocked. Identification and delineation of the ischemic (necrotic) core of the stroke from the surrouding ischemic penumbra (where brain cells are still alive) can help salvage as much of the brain as possible during the stroke treatment.
The CDS Lab at UTA is currently working on the development of data-driven and machine-learning approaches to segmentation of MRI and CT images of ischemic stroke.
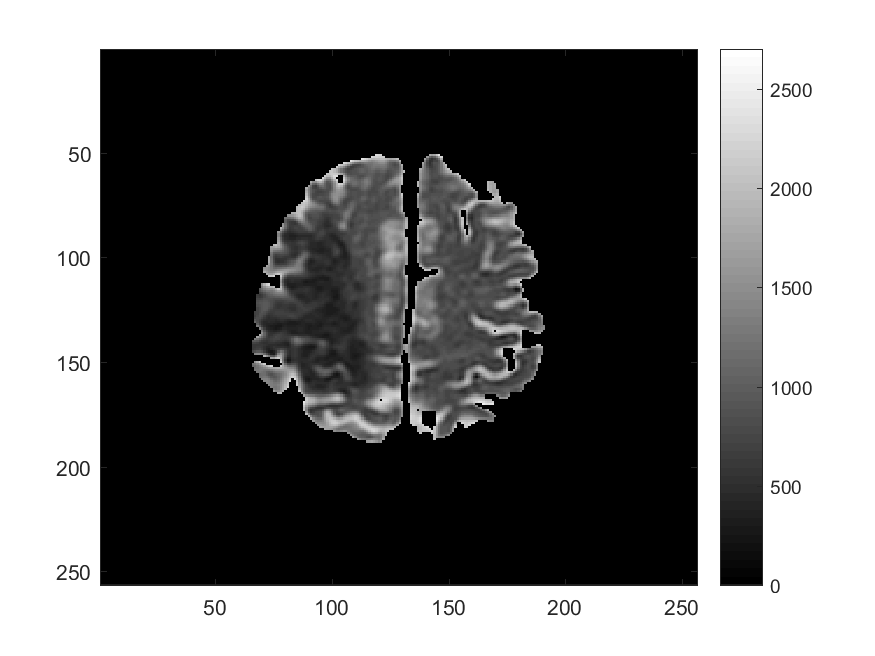
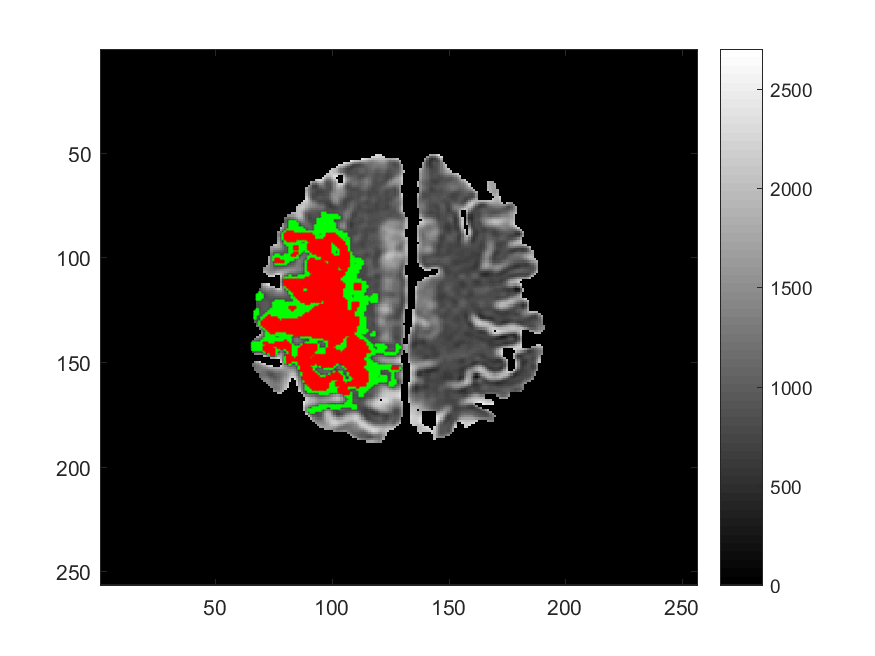
Back to Top
biophysics
Biophysics, Molecular Biology and Evolution
The CDS Lab's research in Biophysics and Molecular Biology focuses on modelling the structure and the Molecular Dynamics of biological macromolecule systems, in particular, proteins. One of the primary goals in my studies was to identify the structural determinants of the squence evolution of viral and eukaryotic proteins. More information on this can be found in the Lab's publications.
The above animation is an example 1.5 [ns] Molecular Dynamics Simulation of the amino acid chains AB of Influenza Hemagglutinin protein at temperature 300 [K]. The simulations were used to measure the effects of amino acid local flexibility on the stability and evolution of proteins. The color coding represent the start of the protein chain A from N-terminus (blue) to the end of the protein chain B, the C-terminus (red).
The simulations were powered by the Stampede's NVIDIA Kepler K20 Graphical Processing Units at Texas Advanced Computing Center (TACC). (Animation courtesy of CDS Lab)
Back to Top
astrophysics
High Energy Physics and Astronomy
In the field of High Energy Physics and Astronomy, the Lab's current research focus is mostly on study of the underlying physics of Gamma Ray Bursts (GRBs), as well as the possible selection effects and instrumental biases that might affect the detection of GRBs by Gamma-ray detectors such as BATSE on board the Compton Gamma Ray Observatory (CGRO), the Burst Alert Telescope (BAT) onboard Swift satellite, and the Fermi Gamma-ray space telescope. These instrumental biases can affect or even create some of the reported empirical correlations among the spectral parameters of GRBs.
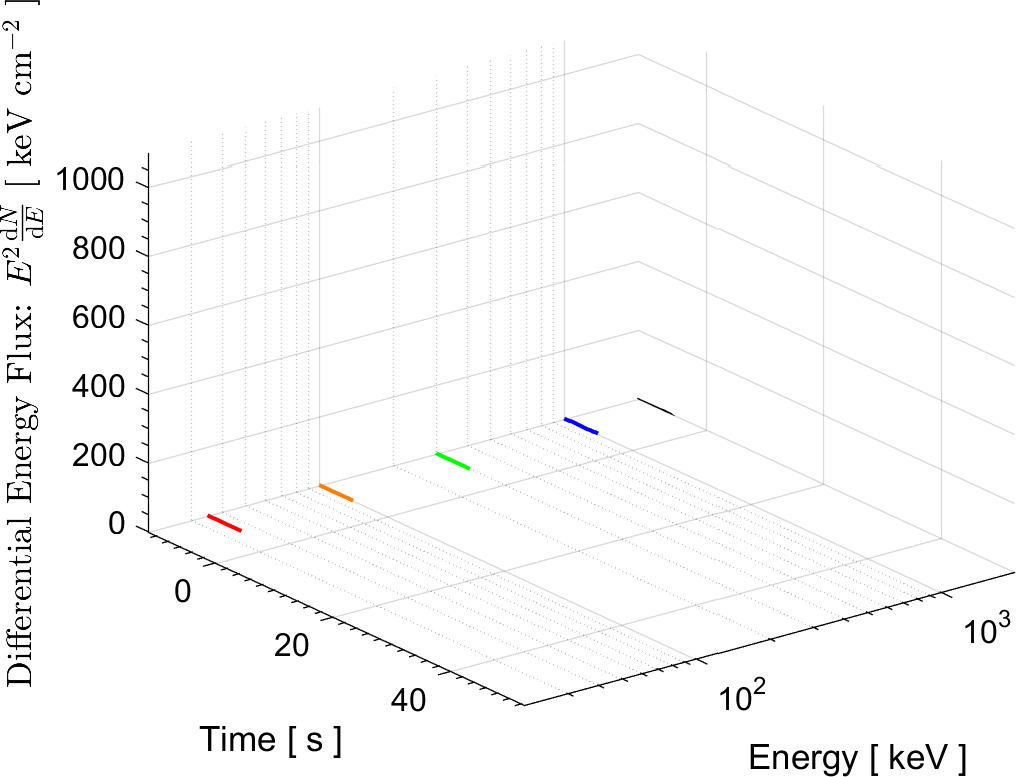
More information on these ongoing researches can be found in the research news below and the Lab's publications.
Back to Top
Statistical Algorithms
Mathematical Modeling, Monte Carlo Techniques
The solution to virtually every scientific inference problem that deals with observational data and the physical world, requires construction of a mathematical objective function, which will have to be then minimized or maximized in order to obtain the most plausible solution (or set of solutions) to the inference problem. When dealing with simple models and small amounts of data, this process of finding the extrema of an objective function is rather straightforward. However, when the model contains many variables (or parameters) and the computation of the function values at different points is costly, the simple methods of optimization and integration fail. As the complexity of the problem grows, a new class of sophisticated optimization techniques known as Monte Carlo methods become relevant and popular.
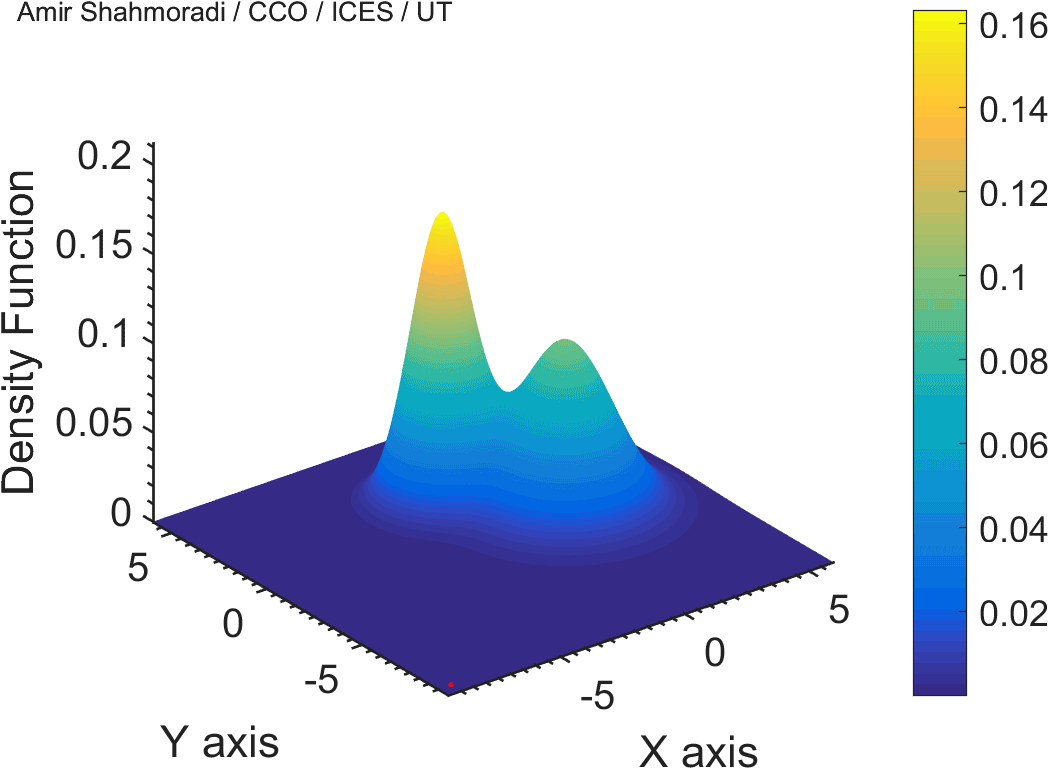
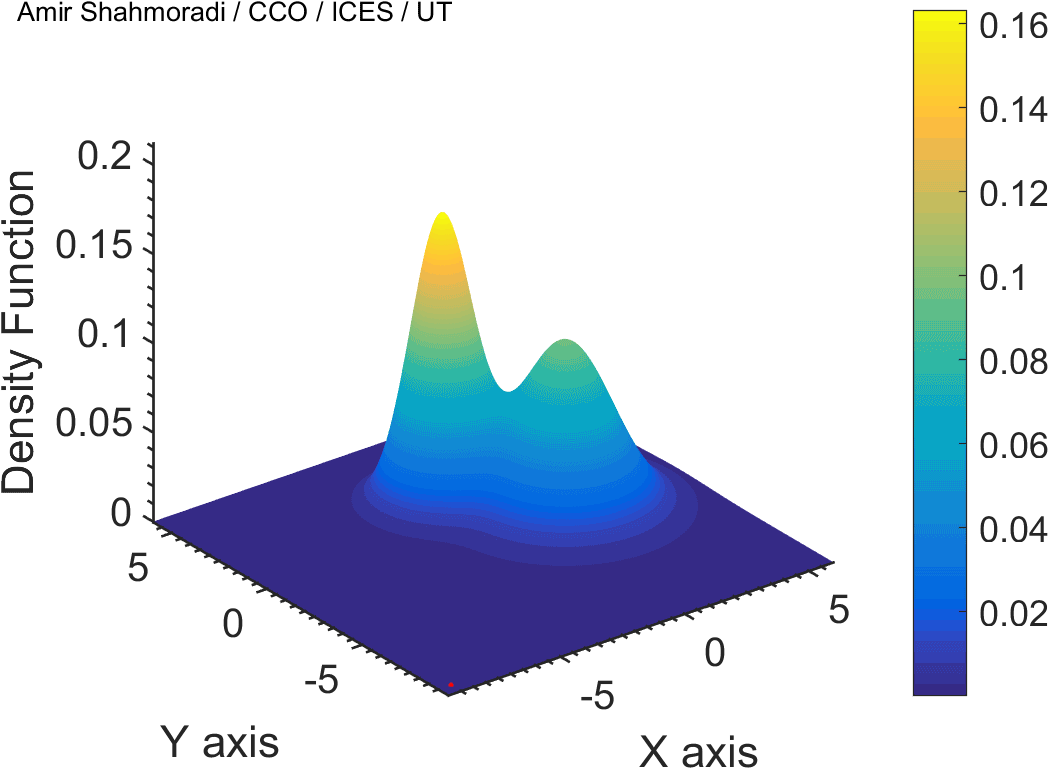
The CDS Lab is currently actively involved in the development of high-performance stochastic optimization and integration techniques, such as automatically-tuned Markov Chain Monte Carlo (MCMC) techniques, Hamiltonian Monte Carlo, Parallel Tempering, Nested Sampling, and many other novel integration and sampling techniques that will be accessible to the public for scientific use.
Back to Top